Artificial intelligence (AI) has captivated the business world, promising revolutionary transformations across industries. From machine learning to natural language processing, AI technologies are celebrated for their potential to automate tasks, analyze vast datasets, and enhance customer experiences. However, the rapid rise of AI has led to an AI hype bubble characterized by weak business cases, bandwagon effects, and inexperience in implementation. Poor business cases and rushed implementations frequently lead to disappointing outcomes and failed projects. Yet, it’s crucial to acknowledge the potential benefits that AI can bring when implemented correctly, such as increased efficiency, improved decision-making, and enhanced customer satisfaction.
Jumping on the AI Train – The Bandwagon Effect
Nobody wants to miss the AI train! However, the bandwagon effect, a significant driver of the AI hype, can lead to hasty decisions. Fearing to lag behind competitors, companies often adopt AI technologies without thorough evaluation. This rush can result in overpromising and underdelivering as companies struggle to align AI with their existing processes and goals. Thus, the potential benefits of AI are put at risk, highlighting the need for caution and careful consideration in AI adoption.
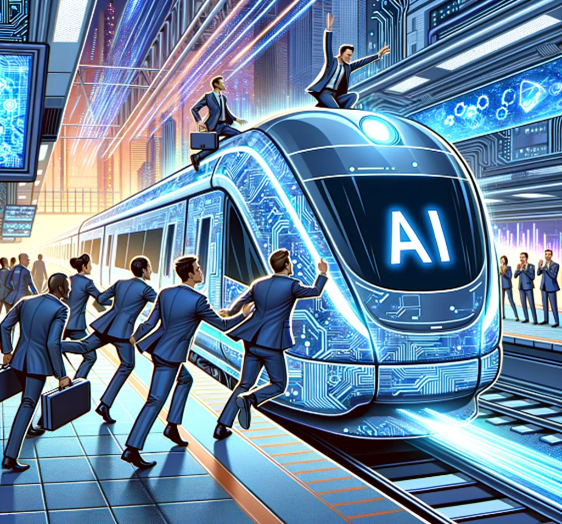
The retail industry, fascinated by AI’s potential to elevate customer experiences, has experienced a surge in AI adoption. Many retailers, eager to leverage data and boost sales while enhancing customer satisfaction, have embraced AI. However, this enthusiasm has sometimes led to rushed AI projects without a clear strategy, resulting in subpar outcomes and unmet expectations. This underscores the importance of careful planning and strategic implementation in AI projects, as the consequences of rushing can be detrimental.
A prominent retail chain made a substantial investment in AI-powered customer recommendation systems, anticipating a significant sales upswing. However, the implementation lacked a robust business case. The data used to train the AI models was incomplete and inconsistent, leading to recommendations that often fell short. This ill-conceived initiative not only squandered resources but also eroded customer trust, a vital asset for any business. This instance serves as a stark reminder of the importance of a solid business case, meticulous AI implementation, and the potential repercussions of neglecting these factors, thereby underscoring their significance in AI projects.
Pressure to Innovate
The current market pressures companies to innovate, causing many to adopt AI technologies without fully comprehending their implications. As businesses strive to be innovative, they invest in AI without clearly understanding how it aligns with their existing processes and goals. This leads to AI becoming a “solution looking for a problem.” Unfortunately, this bandwagon effect has resulted in overpromising and underdelivering as companies rush to implement AI solutions without a comprehensive plan.
Weak Business Cases, the Heart of Failed AI Projects
One major problem contributing to the overinflated hype around AI is the lack of solid business cases. Companies often create business cases based on unrealistic expectations or incomplete analyses that overestimate what AI can accomplish. When businesses fail to establish a solid business case, they risk investing in projects that are bound to fail.
The healthcare industry has seen much hype around AI, promising improved diagnostics, personalized treatment plans, and streamlined administrative tasks. However, implementation issues and weak business cases have led to many setbacks in AI projects in healthcare.
IBM’s Watson for Oncology was expected to revolutionize cancer treatment by providing personalized recommendations to oncologists. While it was an exceptional and innovative concept, the AI system could not meet these expectations due to challenges with data integration. Watson’s AI models often provided inconsistent or incorrect recommendations, leading to technical limitations and a weak business case. As a result, the initiative failed, costing IBM millions and resulting in layoffs in its AI division.
One of the most common flaws in weak business cases is the failure to consider data quality and technical challenges. AI technologies require high-quality data to function effectively, but many companies overlook this aspect. Without a robust data infrastructure, AI projects are bound to encounter issues.
Additionally, weak business cases tend to ignore organizational readiness for AI adoption. Companies often focus solely on the technology without considering whether their teams have the necessary skills and expertise to implement and maintain AI systems. This lack of readiness can lead to costly delays, rework, and even project abandonment. Therefore, companies must assess their readiness levels before embarking on AI projects to ensure they are adequately prepared to implement and maintain AI systems.
Comparing the Past to the Present
There is so much hype surrounding AI, mainly because people lack experience implementing it. Organizations of all sizes have had experience with ERP implementations over the last 25 to 30 years. Some have been successful, others not so much. Implementing AI technologies in organizations today resembles ERP implementations, with notable differences in scope, complexity, technical, organizational skills, and business impact.
Similarities
AI and ERP implementations require significant organizational change, technical expertise, and careful planning. Like ERP, AI projects can face employee resistance due to the fear of automation or job displacement. Both implementations necessitate a clear business case, detailed project management, and significant investment in technology and human resources.
Differences
AI implementations tend to be more experimental and iterative, emphasizing data quality, model training, and algorithmic refinement. They often require specialized data science and machine learning skills, which are in high demand. On the other hand, ERP implementations focus on integrating enterprise-wide systems and aligning them with established business processes. They are typically more structured, with clear deliverables and deadlines.
While ERP implementations have historically struggled with scope creep, customization issues, and resistance to change, AI projects face challenges with high rates of false positives, ethical concerns, and technical complexities. Moreover, AI technology is rapidly evolving, so companies must continually update their systems, whereas ERP implementations generally have longer life cycles with less frequent updates. Both require a strategic approach and stakeholder buy-in to ensure successful adoption and business transformation.
We are reminded of a saying years ago that ERPs should come in a wrapper emblazoned with “Do Not Attempt This on Your Own.” The same can be said today for many companies considering AI.
Barriers to Success
AI projects are inherently complex, involving multiple stages such as data collection, preprocessing, model training, and deployment. Companies lacking experience in these areas face significant hurdles. For example, a financial services company might invest in AI for fraud detection without fully understanding the intricacies of machine learning models. If the models are not adequately trained or validated, they can produce false positives, leading to customer dissatisfaction and financial losses.
Although AI technologies are instrumental in fraud detection for financial institutions, their effectiveness depends on proper implementation and calibration. Missteps in training and validation can lead to systems that produce a high rate of false positives, causing customer dissatisfaction and significant operational burdens.
HSBC’s traditional rules-based approach to anti-money laundering (AML) led to a vast number of false positives, requiring the bank to increase its anti-fraud workforce fivefold since 2012 (Banking Gateway, https://www.banking-gateway.com/analysis/hsbc-financial-crime-ai/. This illustrates the challenge of dealing with poorly tuned AI systems, as false positives drain resources and erode customer trust. Danske Bank experienced similar issues, with 1,200 false positives per day at its peak, with 99.5% of all flagged cases being non-fraudulent (Fintech Futures, https://www.fintechfutures.com/2017/10/danske-bank-turns-to-teradata-for-ai-powered-fraud-detection/). Such high rates of false positives require substantial human intervention to investigate and correct, leading to delays and increased costs.
To overcome these challenges, financial services companies must ensure their AI systems are thoroughly trained and validated to minimize false positives. This requires a comprehensive understanding of machine learning, robust data quality, and continuous model optimization (Fraud Consortium, https://fraudconsortium.org/2024/03/11/revolutionizing-fraud-detection-predictive-analysis-and-ais-role-in-the-financial-sector).
Importance of Technical Expertise
To implement AI successfully, a team with diverse skills, including business domain experts, data scientists, engineers, and design thinking resources – human factors, designers, user experience (UX), and user interaction (UI) experts. If a company lacks this expertise, it often relies on external consultants. However, this approach also has risks if the consultants are not thoroughly vetted. Inexperience can result in flawed models, biased algorithms, and security vulnerabilities, all of which can undermine the success of AI projects.
AI is often used for predictive maintenance and quality control in manufacturing. A manufacturing company may adopt AI to optimize its production processes, but it is essential to consider the complexities of integrating AI into existing systems. If the AI models are not correctly calibrated or tested, they can lead to disruptions in production, resulting in costly downtime and decreased productivity.
Overcoming the AI Hype Bubble
To avoid falling into the AI hype bubble and avoid any potential disasters, businesses can learn from past mistakes by adopting a measured approach to AI adoption. This means addressing the root causes of previous tech hype cycles and applying those lessons to current AI strategies.
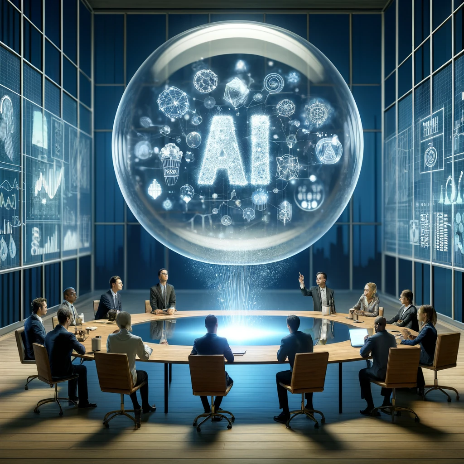
Similar hype cycles, such as those surrounding the dot-com boom or the rise of data analytics, were driven by exaggerated expectations and quick adoption without clear business objectives in the past. To avoid these pitfalls, businesses should develop comprehensive cases with clear objectives and realistic assumptions. This approach involves setting specific goals, defining success metrics, and conducting a thorough cost-benefit analysis—estimating the actual cost—to ensure the investment in AI is worthwhile.
Investing in a skilled AI team and comprehensive project planning is crucial in overcoming implementation challenges. These steps help to address potential blind spots and mitigate risks associated with AI adoption. Involving external experts in the planning process can be valuable, offering additional insights and reducing the risk of inexperience.
It is essential to critically evaluate AI’s potential benefits to avoid the bandwagon effect. Rather than following trends, companies should focus on how AI can solve specific business problems. This strategic approach helps avoid overhyped projects and failed implementations, minimizing the risk of being the next big AI-related disaster.
Businesses should learn from past experiences to recognize the complexity and volatility of AI technologies. The AI hype bubble can lead to severe disruptions if companies fail to consider the long-term implications. By addressing weak business cases, steering clear of bandwagon effects, investing in skilled AI teams, and understanding the actual AI implementation costs, organizations can successfully navigate the AI hype bubble and harness the true potential of AI technologies. To avoid falling into the AI hype bubble and avoid any potential disasters, businesses can learn from past mistakes by adopting a measured approach to AI adoption. This means addressing the root causes of previous tech hype cycles and applying those lessons to current AI strategies.
About Gryphon Citadel
Gryphon Citadel is a management consulting firm located in Philadelphia, PA. Our team provides valuable advice to clients across various industries. We help businesses adapt and thrive by delivering innovation and tangible results. Our services include assisting clients in developing and implementing business strategies, digital and organizational transformations, performance improvement, supply chain and manufacturing operations, workforce development, planning and control, and information technology.
At Gryphon Citadel, we understand that every client has unique needs. We tailor our approach and services to help them unlock their full potential and achieve their business objectives in the rapidly evolving market. We are committed to making a positive impact not only on our clients but also on our people and the broader community.
Our team collaborates closely with clients to develop and execute strategies that yield tangible results, ensuring they thrive amid complex business challenges. If you’re looking for a consulting partner to guide you through your business hurdles and drive success, Gryphon Citadel is here to support you.