Finding Clarity Amid the Static
Imagine a HAM radio operator in a weathered shack, the air thick with static as a storm rages outside. With practiced precision, the operator twists the dials, filtering out interference to capture a faint but vital signal—a voice delivering urgent news through the chaos. The parallel is striking for business leaders navigating artificial intelligence (AI). With its potential to permeate corporate strategies, investment decisions, and public discourse, the AI signal offers hope. Yet, the consequences of noise, in the form of hype, misinformation, and misapplication, can obscure this promise. The task isn’t merely to adopt AI but to tune it with precision and intent, isolating the practical, transformative value signal from the noise that drowns it out.
The stakes are high. Bain projects global spending on AI products and services to reach between $780 billion and $990 billion by 2027. However, BCG projects only 15% of companies have achieved significant financial benefits from AI investments.
This disparity underscores the need to prioritize clarity over clutter, a challenge encapsulated by the Signal-to-Noise Ratio (SNR) engineering concept.In the context of AI, the signal represents actionable insights that optimize supply chains, enhance decision-making, and create competitive advantages. Accenture highlights that AI empowers businesses to streamline supply chains with minimal human intervention, boosting competitiveness, agility, and resilience while driving more profound customer satisfaction. Additionally, AI algorithms have revolutionized operations management by improving forecasting accuracy, optimizing routing, and enhancing decision-making processes. These advancements underscore AI’s potential to transform data into strategic assets, driving industry efficiency and innovation.
It’s not enough for enterprises to adopt AI blindly; they must refine their approach to extract genuine, high-impact insights from AI-driven models. When effectively tuned and aligned with business strategy, AI has the potential to reshape industries and outcomes. Just as a HAM radio operator skillfully isolates a critical transmission from background static, leaders must master AI tuning to transform potential into performance.
Defining Signal and Noise in AI – Clarity vs. Chaos
AI’s signal manifests most clearly when its applications solve genuine problems with precision and measurable impact. Agentic AI, an emerging paradigm characterized by autonomous reasoning and adaptability, exemplifies this capability. Unlike traditional machine learning models, which rely heavily on pattern-matching under human supervision, Agentic AI systems independently reason and dynamically adapt to evolving conditions without continuous human oversight. AI’s signal is most evident in applications that address real-world problems with precision and measurable impact. For instance, Siemens has been at the forefront of integrating AI to enhance energy infrastructure. In 2025, Siemens showcased industrial AI and digital twin technology innovations, aiming to optimize operations and improve efficiency across various sectors.
Similarly, Enterprise AI has proven instrumental in complex decision-making processes. A 2024 Accenture study revealed that companies with next-generation supply chain capabilities, often leveraging AI, achieved 23% greater profitability compared to their peers. These organizations are six times more likely to integrate AI and generative AI across their supply chains, underscoring the technology’s role in enhancing efficiency and resilience.
Conversely, noise in AI encompasses distortions that erode trust and efficacy. Generative AI’s “hallucinations”—confidently presented yet factually incorrect outputs—are a glaring example. While specific studies quantifying these inaccuracies are ongoing, the phenomenon has raised concerns about the reliability of AI-generated information. Beyond technical pitfalls, media hype amplifies the chaos. For instance, sensational claims about AI’s potential to replace most jobs by 2030 contrast with more measured analyses, leading to misconceptions and misplaced fears. Such inflated narratives can drive executives to pursue illusions over outcomes, diverting resources from practical applications. Other forms of noise include data quality issues, lack of transparency in AI models, and misalignment of AI with business goals.
Furthermore, the Gryphon Citadel concept of “data dementia” warns of the self-reinforcing degradation of AI outputs. As generative models train on their content, they risk losing fidelity to real-world data, resembling “a snake eating its tail.” This degradation jeopardizes long-term reliability, especially as web-scraped datasets become increasingly contaminated with synthetic data. The HAM radio analogy illustrates this divide – the signal is the clear transmission cutting through the ether, while noise is the crackle of overlapping frequencies. Enterprises must fine-tune AI strategies to enhance clarity, requiring technical rigor, strategic alignment, and a critical eye on emerging risks.
The Signal-to-Noise Ratio in AI - A Framework for Precision
In engineering, the Signal-to-Noise Ratio (SNR):
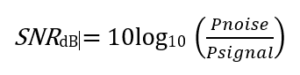
quantifies how distinctly a signal rises above interference. In Artificial Intelligence (AI), the Signal-to-Noise Ratio (SNR) is a pivotal metric for evaluating system effectiveness. A high SNR indicates that an AI system delivers reliable, business-aligned insights, whereas a low SNR suggests the presence of confusion and increased costs due to inaccuracies and inefficiencies. At Gryphon Citadel, we have developed a tailored metric for AI, adapting the traditional SNR concept to measure clarity over chaos. Our formula frames insights as the signal—valued for accuracy, relevance, and reliability—and misinformation as the noise, encompassing errors, hallucinations, and biases. This approach aligns with industry observations that top-performing firms, achieving substantive revenue gains from AI, anchor their success in three pillars.
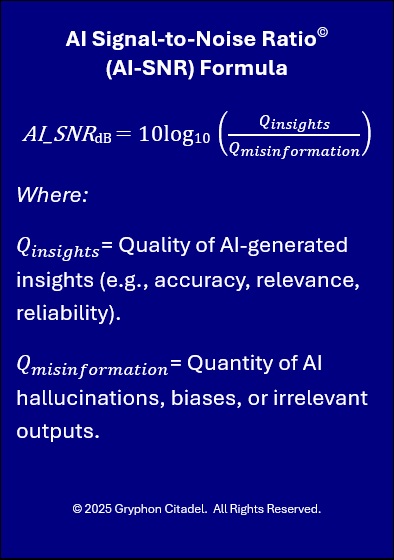
First, data quality and integrity underpin signal strength. Data quality is fundamental to the strength of the signal in AI systems. IBM estimates that poor data quality costs the U.S. economy approximately $3.1 trillion annually, affecting productivity and increasing maintenance costs. This underscores the importance of clean, curated data in driving precision and enhancing the quality of AI-generated insights. Second, explainability and transparency in AI models are crucial for fostering trust and reducing misinformation. A study highlighted that AI models lacking transparency can lead to biases and ethical concerns, particularly in medical diagnostics. Conversely, transparent AI models, which detail their logic and decision-making processes, are more likely to be trusted and adopted by professionals, thereby reducing errors and building user confidence. Third, business alignment and utility connect AI initiatives to their purpose. Clear business objectives ensure the technology serves its intended purpose and delivers relevant outcomes. Gartner research indicates that poor data quality costs organizations an average of $12.9 million annually, often due to a lack of clear key performance indicators (KPIs) and alignment with business goals. In contrast, successful AI projects set specific goals that sharpen insights and drive measurable outcomes.
This framework mirrors the craft of a HAM radio operator – just as tuning a receiver filters out static to isolate the intended voice, Gryphon Citadel’s metric prioritizes insights over noise. For example, a transparent, data-driven AI system can significantly reduce fraudulent activities, resulting in substantial annual savings—a high-SNR triumph. Conversely, unvalidated models may increase false positives, causing operational inefficiencies—a low-SNR misfire.
AI_SNR isn’t theoretical—it’s the difference between strategic wins and operational setbacks. Enterprises must calibrate relentlessly, leveraging tools like Gryphon Citadel’s formula to ensure AI amplifies value, not noise.
Sources of Noise and How to Silence Them
Noise in AI isn’t a minor annoyance—it’s a strategic liability that can derail well-intentioned efforts. Three sources dominate, each with a proven remedy to restore clarity.
Feature set noise arises when models process irrelevant or unstructured data, leading to distorted outcomes. A 2024 McKinsey report found that 70% of enterprises face “application overload,” where an excess of AI tools creates inefficiencies rather than improvements. To address this, Deloitte recommends streamlining datasets to core variables, which has been shown to enhance forecast accuracy by up to 25%. Careful data selection reduces noise, thereby improving focus and performance.
Hallucinations in generative AI present a subtle yet equally damaging threat. A McKinsey survey highlighted inaccuracy as a significant risk, with 44% of organizations experiencing negative consequences from AI inaccuracies. To mitigate such risks, incorporating human-in-the-loop validation is essential. This hybrid approach ensures outputs reflect reality, not fiction, enhancing AI systems’ reliability. Our article on Data Dementia underscores a related danger. As AI-generated content floods training data, models risk “data dementia,” becoming unmoored from authentic distributions—a cycle that demands proactive filtering.
Hype-driven missteps, fueled by media overreach and executive overconfidence, complete the trio. A McKinsey survey revealed that 65% of respondents’ organizations regularly use generative AI, nearly double the percentage from the previous survey ten months prior. This indicates rapid adoption may outpace strategic planning. To counteract this, anchoring AI initiatives in phased, measurable goals—such as targeted cost reduction—can lead to consistent successes. This disciplined approach aligns AI deployment with realistic expectations and tangible outcomes.
The HAM operator’s challenge is clear – noise is the crackle that overwhelms communication, but a filter restores clarity. In AI, disciplined curation, validation, and realism act as that filter, ensuring insights shine through the distortions. Enterprises that master this balance turn potential into performance, while those that do not amplify chaos.
Case Studies – High-SNR vs. Low-SNR AI in Action
Real-world applications underscore the critical importance of fine-tuning Artificial Intelligence (AI) systems to achieve high Signal-to-Noise Ratios (SNR), ensuring precision and alignment with organizational objectives.
High-SNR AI Implementation
In 2023, Aioi Nissay Dowa Europe (AND-E) implemented an AI-driven claims processing system that utilized real-time data and transparent algorithms. This integration led to a 120% increase in the detection of fraudulent claims, resulting in annual savings of up to 2% of their capped indemnity spend. This outcome underscores the efficacy of AI in enhancing operational efficiency and financial performance.
Low-SNR AI Implementation
In 2024, Forward, a healthcare startup founded by former Google and Uber executives, aimed to revolutionize primary care with advanced AI-driven “CarePods.” These high-tech setups featured UV lighting and large screens to facilitate self-administered medical tests without direct human interaction. Despite raising over $650 million, including $100 million in a Series E funding round in 2023, the venture encountered significant challenges. Technical failures, logistical hardships, and low user adoption plagued the initiative. Automated blood draws often malfunctioned, and patients faced difficulties operating the pods. Financial strains and slower-than-expected growth led to substantial layoffs and, ultimately, the abrupt closure of all Forward locations in November 2024. This case underscores the complexities of integrating AI into healthcare. It serves as a cautionary tale about the challenges of merging cutting-edge technology with the nuanced demands of medical services.
Aerospace and Defense Sector Insight
These diverse insurance, healthcare, and manufacturing examples converge on a fundamental principle: high-SNR AI systems, built on quality inputs, transparency, and focused objectives, drive superior performance. In contrast, low-SNR AI systems, plagued by inadequate data and opaque processes, often lead to significant losses. Much like a skilled HAM radio operator filters out static to achieve clear communication, enterprises must meticulously tune their AI systems to amplify accuracy and minimize errors, thereby converting technological potential into tangible success.
The Future of AI – Amplifying Signal, Reducing Noise
AI’s trajectory increasingly emphasizes refinement over sheer scale, guided by three key emerging trends. First, Agentic AI—AI systems capable of autonomous decision-making and dynamic adaptation—is projected to deliver significant efficiency gains. In logistics, for instance, AI-driven route optimization enabled UPS to improve delivery efficiency, reducing overall transit times by approximately 25% and demonstrating clear, tangible business benefits of adopting agentic technologies.
Second, tightening governance frameworks will profoundly influence AI implementation strategies. The European Union’s AI Act, effective from February 2, 2025, imposes strict compliance obligations, with substantial penalties reaching up to €35 million or 7% of global annual turnover for prohibited AI practices. This regulation mandates comprehensive bias audits and rigorous ethical oversight, thus compelling enterprises to proactively embed compliance into their AI adoption plans. Early adopters will likely leverage their compliance readiness into competitive differentiation.
Third, the trend toward human-AI augmentation, rather than wholesale workforce replacement, is shaping future operational paradigms. Organizations integrating human insights with machine intelligence are poised to outperform competitors in decision accuracy and innovation outcomes. The World Economic Forum’s ‘Jobs of Tomorrow: Large Language Models and Jobs’ report indicates that business leaders expect 23% of global jobs to change in the next five years due to technological advancements, reinforcing the collaborative future of work.
Yet, significant emerging risks require proactive management. As generative AI becomes prevalent, the challenge of “Data Dementia”—the dilution of AI accuracy due to recursive training on increasingly synthetic content—poses real threats. Synthetic data is projected to constitute as much as 50% of training datasets by 2030, risking degradation in AI output fidelity. Effective mitigation will require robust data governance practices, synthetic data audits, and hybrid training methods blending human and machine-generated inputs.
Analogous to a HAM radio operator transitioning to advanced digital equipment for clearer reception, enterprises must similarly refine their AI systems rigorously. Prioritizing clarity, transparency, and strategic alignment in AI investments enables organizations to convert potential disruptions into strategic advantages, amplifying signal and minimizing noise.
Tuning for Triumph
AI’s potential is vast, but its benefits rely on precision. Like a HAM operator cutting through a storm’s static, leaders must eliminate distractions—hype, hallucinations, and data drift—to enhance the signal: insights that can transform operations and markets. Ground AI in quality data, demand transparency and align it with measurable objectives. Implement Agentic and Enterprise AI diligently, train teams to critically assess outputs, and safeguard against emerging threats like data dementia. The future favors not those who adopt AI with the loudest fanfare but those who intelligently fine-tune it—transforming a cacophony of possibilities into a clear, resonant voice of enterprise value.
For those looking to master this balance, subtle guidance can make a difference. Gryphon Citadel’s insightful perspective provides a gentle push toward clarity, making it a valuable resource for sharpening your AI strategy.
Mark B. Hassenplug, Managing Partner at Gryphon Citadel, contributed insights to this article from his extensive knowledge of technology strategy, AI innovation, and global business transformation. His perspective, informed by his experience as an amateur radio operator, underscores the critical need for executives to separate actionable signals—practical AI solutions—from the pervasive noise generated by media hype and superficial applications.
About Gryphon Citadel
Gryphon Citadel is a management consulting firm based in Philadelphia, PA. Known for our strategic insight, our team delivers invaluable advice to clients across various industries. Our mission is to empower businesses to adapt and flourish by infusing innovation into every aspect of their operations, leading to tangible, measurable results. Our comprehensive service portfolio includes strategic planning and execution, digital and organizational transformations, performance enhancement, supply chain and manufacturing optimization, workforce development, operational planning and control, and advanced information technology solutions.
At Gryphon Citadel, we understand that every client has unique needs. We tailor our approach and services to help them unlock their full potential and achieve their business objectives in the rapidly evolving market. We are committed to making a positive impact not only on our clients but also on our people and the broader community. At Gryphon Citadel, we transcend mere adaptation; we empower our clients to architect their future. Success isn’t about keeping pace; it’s about reshaping the game itself. The question isn’t whether you’ll be part of what’s next—it’s whether you’ll define it.
Our team collaborates closely with clients to develop and execute strategies that yield tangible results, helping them to thrive amid complex business challenges. Let’s set the new standard together. If you’re looking for a consulting partner to guide you through your business hurdles and drive success, Gryphon Citadel is here to support you.
Explore what we can achieve together at www.gryphoncitadel.com